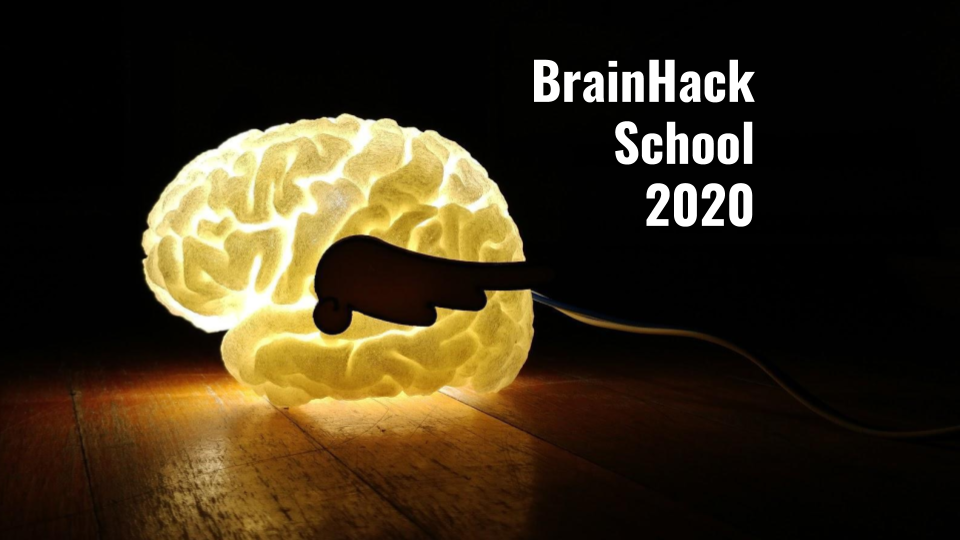
fMRIPrep 101 - Pre-processing fMRI data and extracting connectivity matrices
By Frederic St-Onge
Published on June 12, 2020
| Last updated on
June 28, 2022
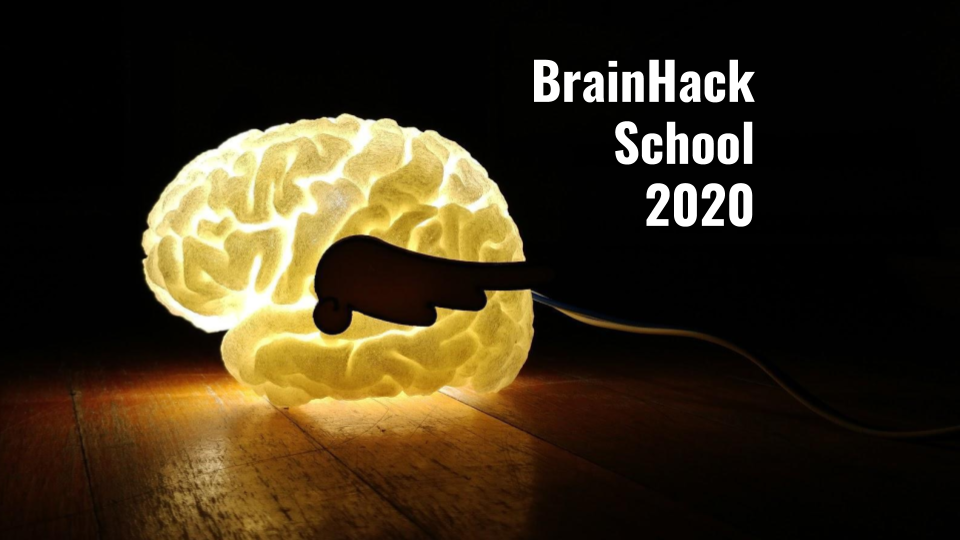
Project definition
Background
Having little experience with neuroimaging and my PhD thesis using fMRI, I wanted to be able to start from raw data (in BIDS format) and learn how to process the data until I obtain derivatives (i.e. connectivity matrices). Based on the current PhD project that I am working on, using various atlases leads to varying results. As such, I wanted to extract connectivity matrices from pre-processed time-series using Nilearn, which comes pre-loaded with several atlases of interest. Finally, as most of my work will involve large cohorts (which might not be feasible to do on a personal computer), I wanted to be able to realize these analyses on a HPC, where resources can be used appropriately. Please note that more information on the project is available on Github.
Tools
The project used the following tools:
- Docker (for bids-validator and fMRIPrep)
- Bids-validator (to validate bids)
- fMRIPrep (to pre-process data)
- Jupyter notebook (for deliverables)
- Nilearn (to extract connectivity matrices)
- Github (to version control and share the project)
Data
The data used is a single, anonymized, subject from the Prevent-AD. We used 2 fMRI visits. Data is not available for reproduction, but more details on the cohort can be found here
Deliverables
At the end of this project, we will have:
- A Jupyter notebook detailing the failed first project.
- A Jupyter notebook detailing the current fMRIPrep project.
- A complete GitHub repo detailing the process.
Results
Github repo
For more details on the deliverables, please refer to the GitHub repo available here.
The tutorial was designed to be: 1) Easy to use, 2) Comprehensive and 3) Hopefully fun!
Conclusion and acknowledgement
In conclusion, I was able to learn a lot of new tools and gain a deeper understanding of fMRIPreprocessing. I was able to create a tutorial on using fMRIPrep that, hopefully, will also serve others learning this software!
I want to thank my instructor, Desiree Lussier, and all the BHS2020 team for allowing me to learn so much in a short span of a month!